Key players insights
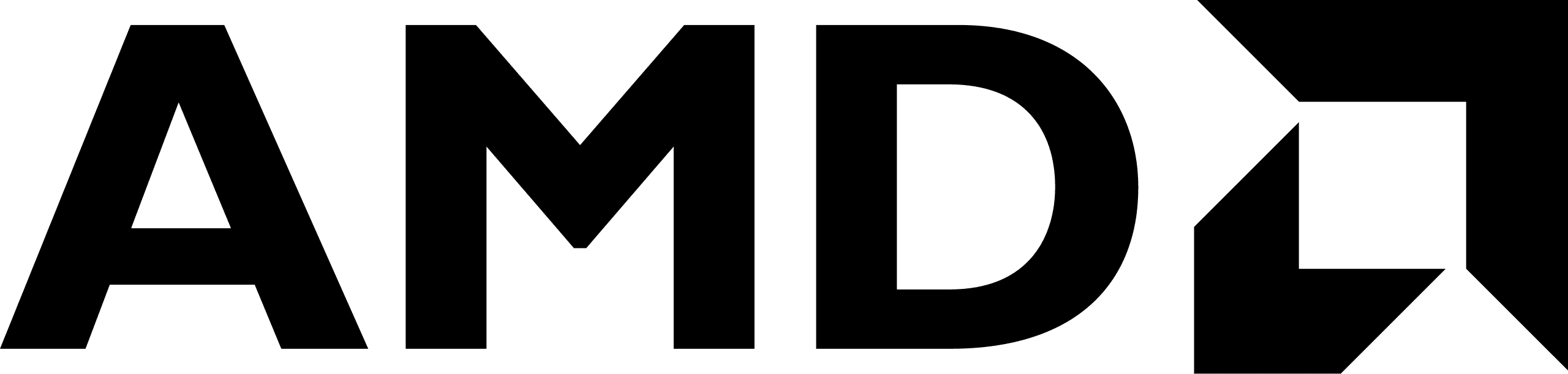
When it comes to AI, it’s foundational to know that training and inference go hand in hand. But while the training of AI is often worked through in the cloud, inference tasks are increasingly migrating to on-premise servers or edge devices. Let’s talk about why this is happening.
To begin, let’s root ourselves in the relationship between training and inference. The training of AI develops models that inference uses to recognize whatever needs identifying. This includes instances like identifying traffic levels on a city’s roadways, transcribing a conference call in real-time, or recommending the next streaming video to watch.
Edge devices connect everyday things to the cloud while processing some data. So why are inference tasks progressively being moved to edge devices? There are several reasons for this, starting with low latency. Simply put, it takes time for raw data to go to the cloud or data center and then back to the edge. For tasks that must be completed in real time, like retail self check-out, speech recognition, and autonomous driving, it can be detrimental for decisions to take too long.
Form factor is important for real-life edge deployments. Fitting hardware into a constrained environment, such as breakroom of your local retail store or under your vehicle windshield is crucial. These environments also have power and thermal constraints where AI inference must fit into a compact PCIe cards or even a credit card size module. Balancing hardware power and cost while maximizing compute utilization for AI inference takes ingenuity.
In order to keep up with the ever-increasing demand of inference workloads at the edge, developers are increasingly leaning into the flexibility afforded by field-programmable gate arrays, otherwise known as FPGAs. FPGAs can be reprogrammed to desired application or functionality requirements after manufacturing. FPGA-based DSAs, or domain specific architectures, can be optimized in real time to deliver compute acceleration for a range of workloads and different forms of AI inference, particularly critical at the edge.
Because AI models continue to accelerate innovation, a one-size fits all hardware architecture is not practical. To maximize the compute utilization, an ideal edge device contains both AI engines and adaptable hardware. This combination will offer the state-of-the-art AI horsepower while allowing DSA hardware to be programmed to efficiently run the specific AI tasks.
For instance, to achieve safe autonomous driving, it’s absolutely critical to have extremely short and deterministic end-to-end latency between sensors detecting an object on the road to AI models to actual actuator operations in the vehicle. When you consider the average human reaction takes a few hundred milliseconds before stepping on the brake pedal, the goal of AI infused autonomous cars is to react even faster, in 100 MS or less. On our roads, where safety is paramount, AI hardware has to process complex AI inference while processing computations for sensor and actuators with very low latency.
While AI innovation accelerates rapidly, AI hardware must adapt efficient domain-specific architectures to meet the challenging requirements of edge deployment. And we are just getting started!
To learn more, tune into Nick Ni’s, Sr. Director of Data Center AI and Compute Marketing at AMD, Keynote speech on February 10th 10:05 to 10:30 on the track AI Strategy at the World AI Festival Cannes.