Key players insights
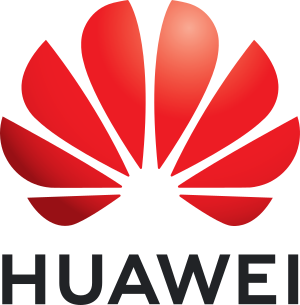
Q: Thomas Weber, you will give a Keynote speech at WAICF on February 9th on “Ultra Large AI Models improving the end-to-end development life cycle”. Can you give us a small preview?
TW: In 2019, Gartner predicted that through 2022, around 85% of use cases leveraging AI techniques would fail. Well, here we are and although I wouldn’t be so bleak, the rate of failure of AI projects is definitely high. Given that AI has only recently become a clear priority for businesses, these numbers are not actually that surprising but it shows a real need for best practices.
Most companies venture into AI with very clear goals in mind – accelerating their time to market being the most notable one – but no clear roadmap of how to implement their project in a structured and timely manner. Data scientists, data architects, database administrator and other technical specialists are brought in alongside experts with actual domain knowledge and together, they have to set up an infrastructure that is capable of operating AI, they have to find the right data, make the right decisions on feature engineering and check which factors to incorporate into their AI models properly. It can become quite complex very quickly. One way to avoid under-delivering is to rely on a solid framework that will organizationally and technologically orchestrate all critical factors into an efficient, valuable and scalable project.
Q: According to you, what are the top best practices in AI project management?
TW: First, make sure you have the right people on your team – a data scientist within a large company can´t run a large scale project alone. Support from the Infrastructure and domain-specific lines of business is always required. Second, make sure the expectations are focused on the main target – have one goal at the beginning, not 10. It’s good to make new findings and have ideas, but first solve the actual issue and then utilize the findings later. Third, try to show the plan you made, the path to success in an easy understandable high-level way – most people don´t know what AI is really like to work with nowadays. The plan should focus on creating fast results and involving the management early.
These might seem somewhat obvious to a lot of people but it takes discipline and focus to maintain these practices for weeks or months, when you feel pressured to prove ROI and determine business value.
Q: How does Huawei help companies handle this complexity? What is your contribution?
Recently, language models like GPT-3 and ChatGPT have been making headlines across the globe and creating a lot of excitement around AI for their extraordinary capacity to perform tasks and generate human-like text, from debugging code to writing songs and poetry. But their extraordinary capacities come at a price: scale. GPT models made a leap forward compared to other models thanks to the staggering size of the dataset they are pre-trained on, definitively establishing the trend for bigger and bigger AI models. While the never-ending increase in the size of AI models leads to better performance, it also introduces new challenges of complexity.
Huawei believes that, because the future of AI is one we will all share, it’s important to make AI models affordable, valuable and easy to implement. Providing inclusive AI is one of Huawei’s core goals in developing a comprehensive AI strategy and full-stack, all-scenario AI portfolio. More particularly, one of our focuses has been to build technologies and frameworks that simplify and automate the most time-consuming tasks related to pre-training large AI models, from preparation to labelling, fine-tuning and adjustment. It provides our customers with a generous head-start for their AI projects, allowing them to see the benefits quite quickly and achieve a ROI within 1 to 3 months.
There is so much more to say about the future of AI models. I’m looking forward to discussing it with you in Cannes.
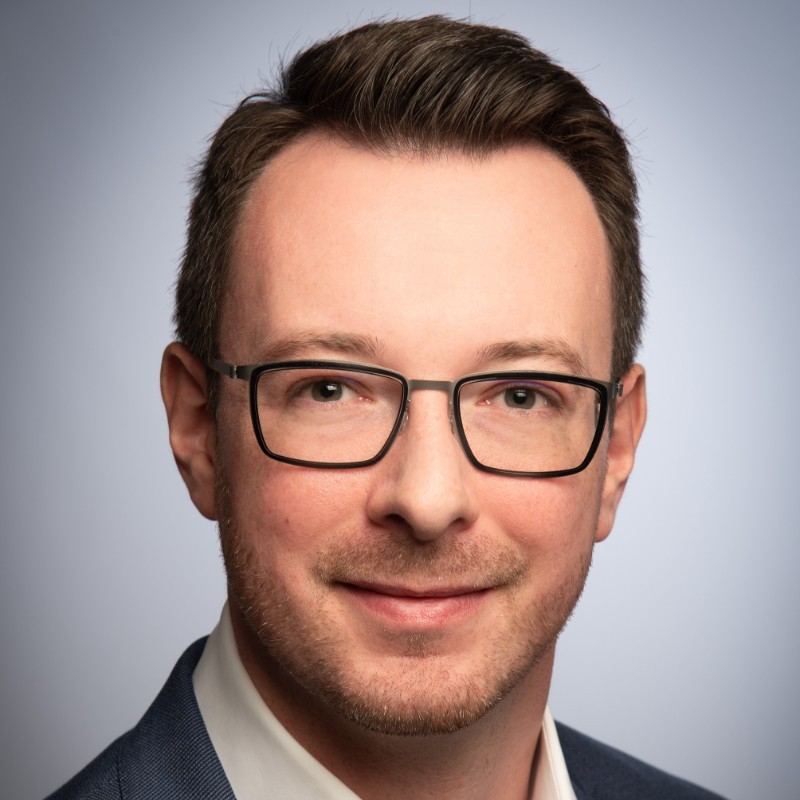