Key players insights
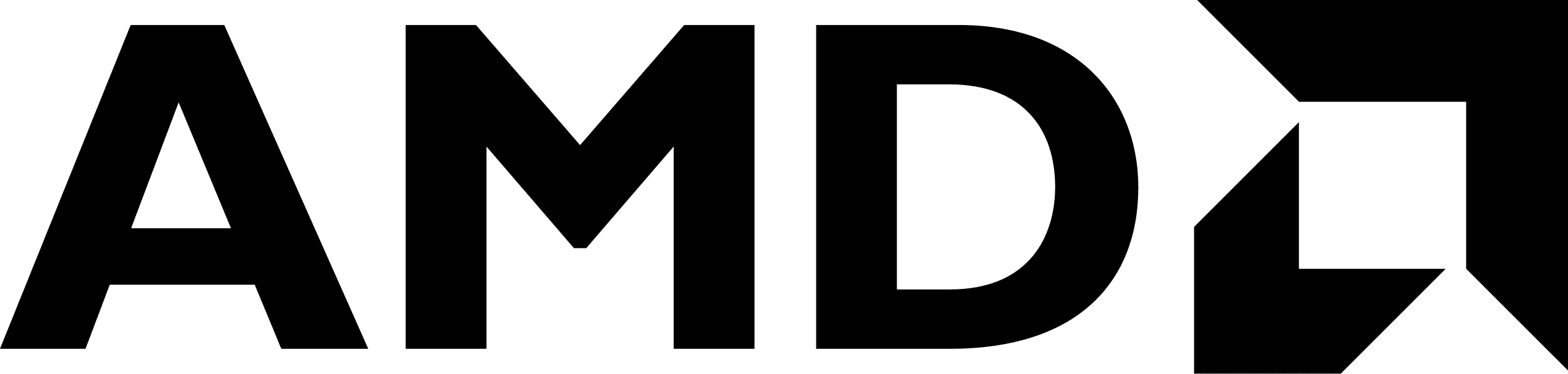
AI is becoming increasingly pervasive thanks to its rapid progression and relative ease of access. In order to continue to achieve advancements in AI inference, we must look at how growth can be made in training. To talk about the current and future states of AI training, let’s start by thinking about pervasive AI in a more tangible context.
AI is very likely integrated in more parts of your life than you may have ever imagined. We’re surrounded by intelligent connected devices. Our homes, our vehicles, our offices, and even our infrastructureare becoming increasingly sophisticated. But while it’s incredible how quickly AI has become pervasive, it still has a long way to go. At this point in time, AI innovation is gated by how quickly you can train your model with ever increasing real-life data.
The training of AI usually takes place in enterprise data centers or in the cloud where many high-powered servers, hardware accelerators, and high-speed networking operate together within a workload. Despite the massive infrastructure, it still takes many hours, even days, to train a single model. Take into account some of today’s largest language models. In the last four years, the size of language model parameters grew by almost one thousand times – from around one-hundred million parameters to nearly two-hundred billion.
It’s also important to note the cost of pervasive AI is an exponential rise in the data-processing and energy efficiency requirements placed on the semiconductors that power these devices. Even the most advanced data center is hitting physical limitations on power supply and thermal, preventing further scaling of hardware resources.
In order to implement the latest and greatest in AI functionality, you need hardware that can keep up. To shorten AI training time and combat hitting power limitations, GPUs are becoming increasingly power efficient, with horsepower reaching Peta (1,000,000,000,000,000) Floating-point Operations per Second and populating with the most advanced High-Bandwidth-Memory (HBM) with Tera-bytes per second of external memory bandwidth in a single GPU. Because today’s largest models simply do not fit into a single GPU, GPU-to-GPU connections are also reachingTera-bytes per second range with extremely low latency to enable scaling the training across many GPUs with minimal overhead.
Ultimately, the answer to progress in AI lies in the hardware that powers that progress. Together, these innovations in hardware will help bring down power requirements in data centers, allowing more training to be executed and enabling rapid advancement in AI models.